Executive Summary
- Training large language models (LLMs) like ChatGPT from scratch requires significant resource usage. For instance, ChatGPT 3’s training consumed energy equal to a year’s usage for 1,000 U.S. households. An arms race amongst the big players for ever more powerful models means the energy requirements are set to expand further.
- Rather than training new models, companies can build on top of a foundation model like GPT, adapting models to specific data. Techniques like retrieval augmented generation and fine-tuning are available through Snowflake Cortex.
- Matching AI with the right use cases conserves resources during the inference phase when the model is deployed into production.
Sustainability Concerns Surrounding AI
The last 2 years has seen artificial intelligence (AI) capture people’s imaginations, with its ability to perform tasks once only possible by humans. However, its popularity has also raised concerns about the resources required to power AI. Sajjad Moazeni, an assistant professor at the University of Washington, estimates that it took the same yearly energy consumption as 1000 US households to train ChatGPT 3 [1], the version that became hugely popular in 2022. Increased competition in the sector and the entry of big players such as Google, Meta and Microsoft is leading to an arms race to create ever more powerful models. This is leading to the energy demands of newer models to become much higher. With sustainability becoming more of a concern for companies, how can the powerful features offered by AI be leveraged without causing undue harm to the planet?
Before answering this question, it’s helpful to get a better understanding of the resource intensity of AI. These concerns are also being raised about one particular family of AI models, namely large language models (LLMs). LLMs are the technology behind popular models like ChatGPT and Gemini. LLM’s high energy consumption is related both to how they work as a technology, as well as the associated build out in infrastructure that’s accompanying the current AI boom.
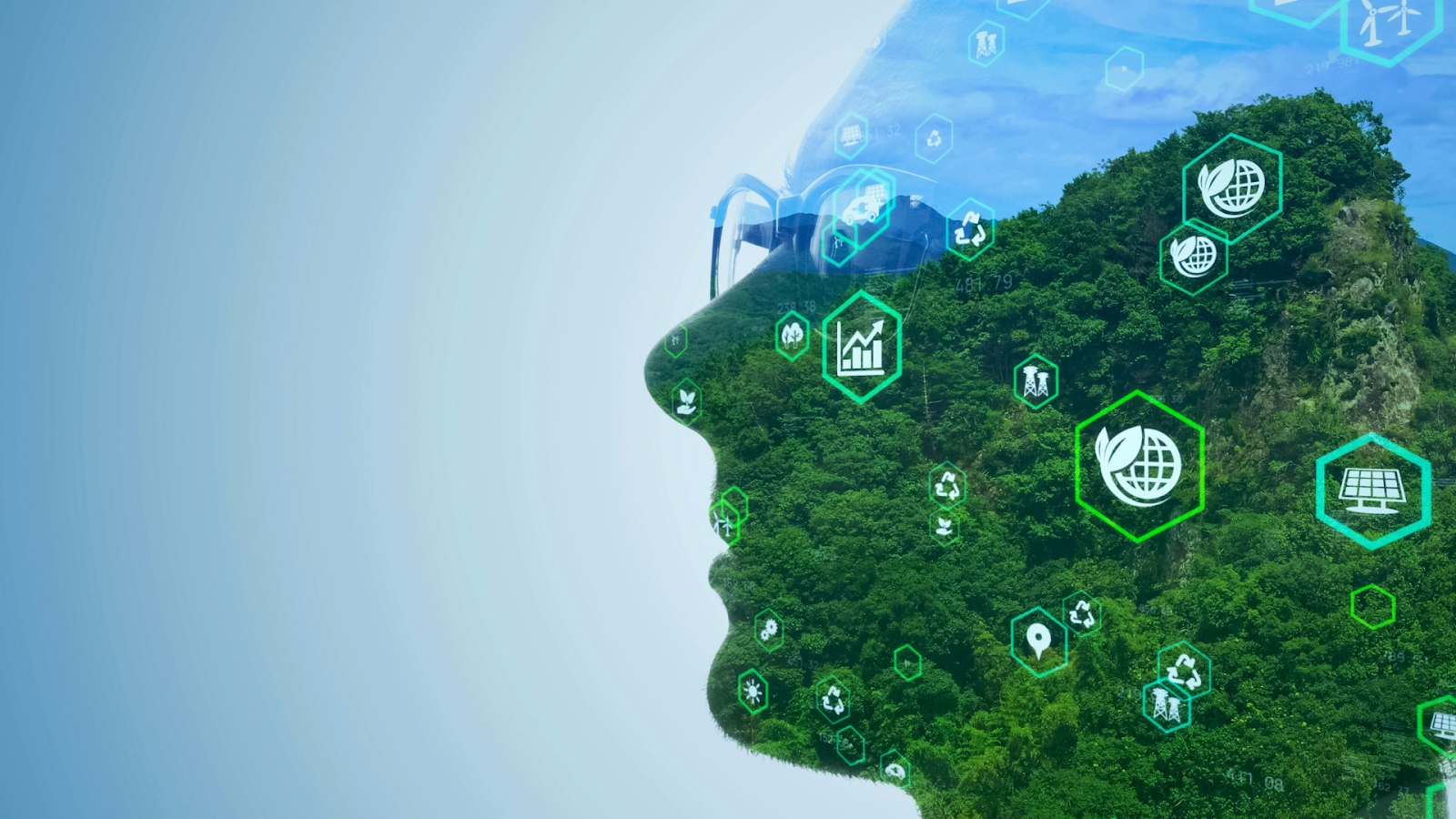
What Are LLM’s?
LLMs work by consuming massive amounts of data, with this data then being processed repeatedly until the LLM learns the connections within the data. Popular models such as ChatGPT are also designed to be general – meaning they need to be able to answer almost any kind of query. In the case of ChatGPT 3, the largest part of its training dataset was 3 years-worth of data scraped from the internet, containing over 570 GBs of text data [2]. The particular architecture used by modern LLMs – the transformer architecture – also requires a lot of processing to be done on the data [3]. The combination of large datasets and intensive processing leads to the training of LLMs being highly energy intensive.
Competition between companies is also leading to an arms race to make more powerful LLMs, with higher energy requirements. The power of an LLM is typically measured by its number of parameters – the number of different factors or data points that are used in deciding the output that an LLM generates. For example, BERT, a model released by Google in October 2018, contained 110 million parameters. The energy required to train BERT was estimated as being around the same as a flight from New York to San Fransisco [4]. By contrast, GPT-3 released by Open AI in 2020, has 175 billion parameters, around 1500 times the number of parameters [5].
Data centres already accounted for 14% of grid usage in Ireland in 2021 – before the AI boom even began [6]. The AI-hype wave is also leading to an acceleration in the construction of data centres, which host the physical infrastructure underpinning AI. Demand for electricity from data centres is expected to grow so much that Google is planning to build small nuclear reactors in the US to power their data centres. It’s not just energy usage which is being impacted. Microsoft’s total water consumption almost doubled between 2020 and 2023, with much of this thought to be related to their scaling up of data centre construction [7].
A Greener Future For AI?
In light of AI’s resource intensity, is it still possible for companies to take advantage of AI in a sustainable manner?
To remove a doubling up on the resource expense associated with training a model from scratch, you can build on top of a foundation model such as Open AI’s GPT-4 or Google’s Gemini. This works on the principle that an AI model trained on a more general set of training data – such as the entire internet in the case of ChatGPT – should also have enough base knowledge to understand new data, without the need for further training. For example, if ChatGPT has had financial documents in the public internet in its training data, then it should already know enough about financial documents generally to understand your organisation’s documents, which it hasn’t been trained on. Certain models are also more adept at performing particular kinds of tasks. For example, Claude 3.5 Sonnet is widely perceived as performing the best on coding related tasks.
Different techniques have been developed which leverage the pattern of using an existing model.
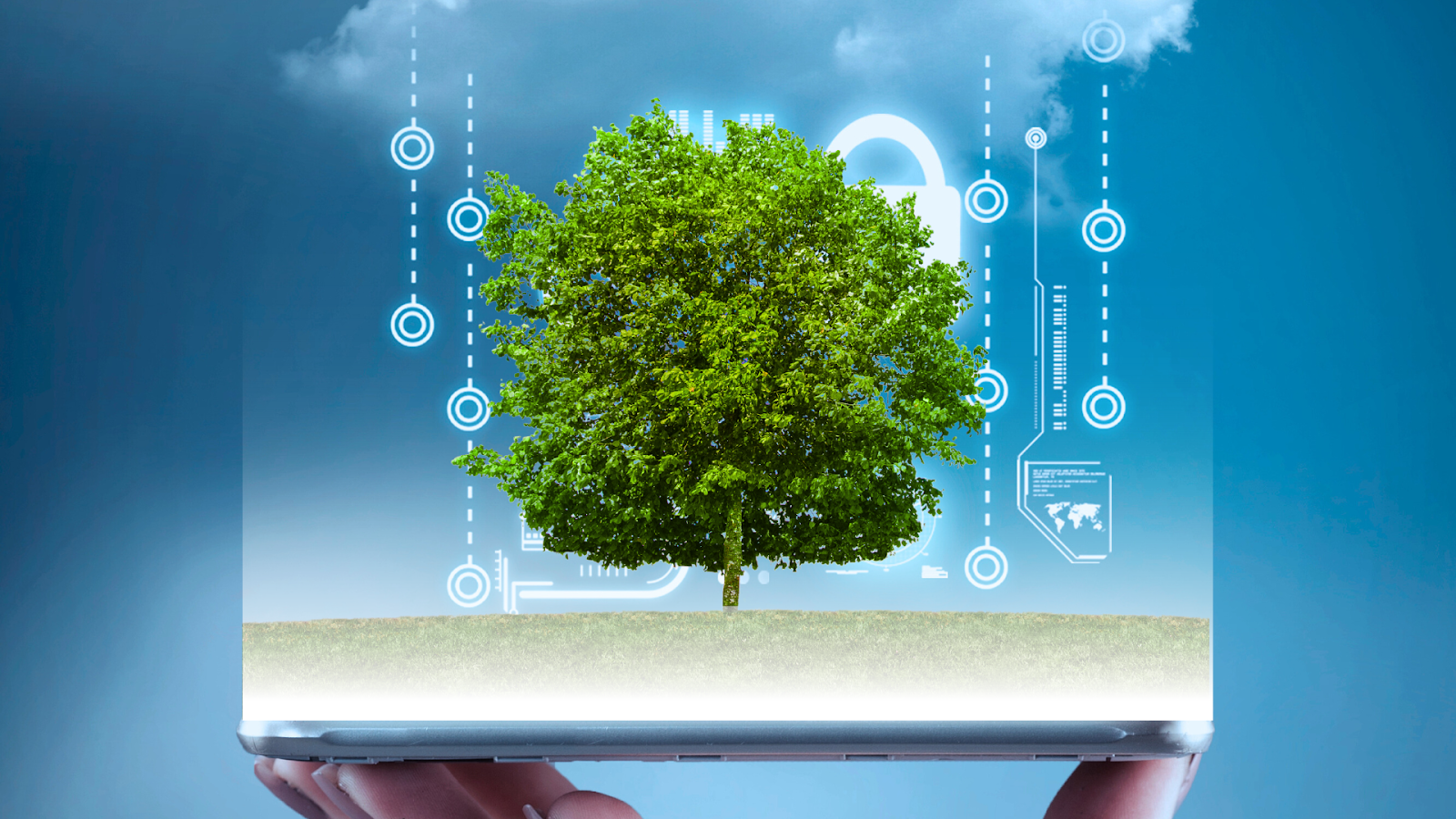
Retrieval Augmented Generation (RAG) involves taking documents, storing them and arranging them based on how similar they are to each other, and then feeding these into an LLM as additional context when interacting with it. This is similar to how you might give ChatGPT additional information when interacting with it via its chat interface, like the person who you’re writing to when generating an email. This allows an LLM to give more specific answers which are more relevant to your organisation. Snowflake currently offers RAG through its Snowflake Cortex Search service.
Fine-tuning involves modifying the internal workings of a foundation model, which can help to achieve more accurate, consistent and faster results when compared with RAG. While fine-tuning is more technically complicated than RAG, Snowflake also offers a fine-tuning service to make this easier.
Although the training phase is the main area of concern for energy usage, the so-called inference phase is also associated with higher energy costs when compared with non-AI approaches. The inference phase is the phase in which a model is given a question to answer. One way to reduce usage on this phase is to be selective about your use cases for AI. Hype waves are typically accompanied by FOMO. While it’s natural to want to make use of a new and exciting technology, it’s important to start with a use case in mind, and then judge whether an LLM would be the appropriate solution. For example, if you wanted to create an application that summarises large volumes of text and make it more understandable to an end user who knows their domain well, then an LLM would be an ideal candidate. It might be less appropriate to apply an LLM to a use case where there’s a high degree of risk created by the LLM hallucinating (making stuff up), such as giving medical advice. Being diligent about how you apply AI can therefore reduce your operational risk, while also helping your organisation to operate more sustainably.
There’s little doubt that AI is leading to increased energy and resource usage. But by building on top of a foundation model and carefully selecting appropriate use cases, it’s possible to make use of AI in a more sustainable manner. This doesn’t just make more sense for the planet, there’s a much better business case for this too.
Sources/Further Reading
[1] Q&A: UW researcher discusses just how much energy ChatGPT uses
[2] Language Models are Few-Short Learners
[3] Hugging Face: How do Transformers work?
[4] Energy and Policy Considerations for Deep Learning in NLP
[6] Central Statistics Office: Data Centres Metered Electricity Consumption 2021
[7] Wired: AI’s Energy Demands Are Out of Control. Welcome to the Internet’s Hyper-Consumption Era